Start Time: 15:00 January 1, 0000 3:46 PM ET
International Business Machines Corporation (NYSE:IBM)
Bank of America Securities Global A.I. Conference 2023
September 12, 2023, 15:00 PM ET
Company Participants
Rob Thomas – SVP Software & Chief Commercial Officer
Conference Call Participants
Wamsi Mohan – Bank of America
Operator
Ladies and gentlemen, the program is about to begin. A reminder that you can submit questions at any time where the Ask Questions tab on the webcast page. At this time, it’s my pleasure to turn the program over to your host, Wamsi Mohan.
Wamsi Mohan
Thank you so much. Good afternoon, everyone. Thank you for joining us on day two of BofA Global AI Conference. I’m delighted that all of you could join us here today. I’m especially delighted to welcome Rob Thomas from IBM for this session. Rob is Senior Vice President of Software and Chief Commercial Officer at IBM. So he leads all of IBM software business including product design, product development, and business development. And in addition, Rob has total responsibility for IBM revenue and profit, including worldwide sales, strategic partnerships and ecosystem. And I feel delighted to welcome Rob because every time I speak with him, I learned something new and walk away a tiny bit smarter. But there’s this ocean of knowledge that I love to tap into and I appreciate the opportunity. So Rob, thank you so much. Welcome.
Rob Thomas
Wamsi, great to be with you and thank you for having me. I appreciate all that you all do.
Wamsi Mohan
Thank you, Rob. I know that you have some slides that you’d like to go through. So let me turn it to you to maybe talk about AI from the IBM context.
Rob Thomas
Sure. I thought I would give a little perspective about where we are and then we’ll leave ample time for questions as well. As I had mentioned in one of our previous discussions, Wamsi, our investment in generative AI goes back to 2020. At that time, Arvind had talked about today’s IBM being hybrid cloud and AI. We’ve talked a lot about Red Hat. We have great momentum with Red Hat. AI was the other piece. And we haven’t talked about that as much until this year, because we really spent three years building a product. And it started with a massive investment and infrastructure.
So we could do training on it when at a time was early in the transformer experimentation what became generative AI and large language models. We then announced watsonx back in May at our Think conference. We’ve had a number of beta clients since the start of the year. And now that watsonx is generally available, I’d say we have a lot of learning in terms of what’s happening with clients and where we’re going to head with the product. So I thought I’d spend a few minutes to kind of share all of that. And then we can have a discussion.
If we go to the next slide, I do think this starts at least for IBM with enterprise data. We’re not trying to be a consumer engine. We’re not trying to just focus on scraping the web to build models. We are trying to deliver generative AI for enterprises, which is actually quite different. So if you think about foundation models and building them, you have to start with what is the data that you have.
And I think this largely informs our strategy, because if you think about the last three years, the models that we are building for IBM were based on the datasets that we knew best. We have great models based on the datasets we have on code and programming languages. We have seven years plus of experience on natural language processing. We started to incorporate IT data, sensor data. In some cases, we partnered with others, in one case, NASA around geospatial. But the thing I want everybody to think about is just the opportunity that exists for enterprise data. And that’s why I call this the opportunity of a lifetime.
It’s very different than consumer. We are grateful for everything that has happened with ChatGPT because it put excitement in every CEO’s mind and every Board of Directors that there was something here. To some extent that did a lot of our marketing for us out of the gate, which we appreciate. Our focus though has been B2B what we do best and how we leverage the promise of the transformer architecture in generative AI for businesses.
We go the next slide. What we announced at our conference in May was really focused on the piece in the middle call it AI and data platform, watsonx. But there’s actually much more to the story in terms of what we’re doing. So I thought just spending a moment on what is the generative AI tech stack that we are investing in. You start from the bottom, it’s about open source that we deliver through Red Hat OpenShift AI.
PyTorch I’d say is an emerging standard and maybe even emerging is understated. I think PyTorch has massive momentum, so committing, contributing to that, incorporating that plus other open source libraries, things like Ray and to OpenShift AI. And this really gives us call it a developer centric or a bottoms up go-to-market for how we’re delivering on AI. Next, you have data services. Most people don’t really think of this as core to an AI strategy. But I can tell you now with nine months under our belt in terms of intensive client work, data fabric, organizing, managing, delivering trusted data becomes pretty essential to any AI project.
Then you have watsonx. That is the core platform. I’ll go into a bit more detail on what that is in a moment. We’re now in the process of delivering a software development kit or SDK for ecosystem integrations. We’ve talked about how SAP was one of the earliest adopters of watsonx integrating as their AI platform. That’s because we’ve made APIs and software development capabilities available to ISV partners.
And last and certainly not least is AI systems. And this is perhaps the most approachable part of the tech stack for any company because it’s really designed in the language of a business user. We have watsonx Assistant, Orchestrate, Code Assistant. I’ll get into a little bit of where this is going. But when you think about generative AI for IBM, this is the tech stack. And yes, we also bring consulting services with the center of excellence that we’ve announced around IBM consulting supporting this.
We go to the next slide. So the last nine months, we have centered in on three use cases. And this is largely based on a lot of trial and error, talking to clients. And I would say confidently at this point, these are the use cases that are not only relevant to nearly every business in the world but the ROI is clear. And we hosted a group at the at the U.S. Open tennis just over this past weekend. And talking to all the CEOs in that group, I think a common refrain was we’ve been doing a lot of experimentation. Now it’s time to get an ROI. And that’s what I really like about what we’ve learned in this process on use cases, because we can deliver these in a pretty seamless fashion.
So number one is around talent. And I would even broaden this a bit to say automating any repetitive task, generative AI is incredibly good at making predictions on tasks that are repetitive in nature, because by definition, if it’s repetitive, doing the prediction and getting accuracy is going to be a lot easier. We see 40% improvements in productivity. HR has been one that we spent a lot of time on, and I’ll talk about why in a moment when I talk about the IBM deployment of this use case for HR.
But like I said, this could generalize beyond talent and HR into things like finance, procurement, supply chain, you can imagine a lot of different ones. The main generative AI tasks here are classification and then content generation. That’s what underlies this. The product that we use for this back to that assistance layer is called watsonx Orchestrate. Orchestrate is basically a platform for building digital skills and then having those codified in generative AI with large language models.
Next is customer service. We’ve been in the market with what is now watsonx Assistant for over five years. What’s different here is when you bring large language models, generative AI, the kinds of capabilities you see here, retrievable augmented generation, summarization, classification, accuracy skyrockets. We’re now seeing 70% plus containment in call center use cases. That means when somebody calls in with a question or types in with a question, in 70% of the cases if you’re using watsonx Assistant, it never has to touch a human. It’s just automated. You can see how that would pretty quickly generate an ROI for our clients.
I’d say customer service is second. Third is app modernization. And we’re seeing a 30% productivity gain in application modernization, specifically around code. And this is delivered through watsonx Code Assistant where for the early work we’ve done around Ansible, we are now seeing 85% code acceptance. So that means 85% of the time that watsonx is recommending code to a developer, they are accepting it and they go on their way. That’s how you get to 30%. It’s pretty simple.
If the code is being accepted, you can drive massive productivity quickly. We recently announced the tech preview what will soon be a general availability of watsonx Code Assistant for Z or the mainframe. And we’ve got to the point now that we have a 20 billion parameter, 1 trillion plus token model for code, which is proving to generalize very well. And so we see this as just the start as we can bring this to other programming languages. So I would say learning for nine months, really excited about these as proven use cases that leverage generative AI and have a clear ROI.
We could go to the next slide. Just to reorient again around what is watsonx. The platform itself has three main capabilities. First is watsonx.ai. This is where you can train, tune, validate, deploy AI models. Think of this as the builders’ studio. And we make IBM models available. I talked about IBM models based on enterprise data. We’ve also partnered with Hugging Face and recently invested in their most recent round as well to deliver basically the world’s largest selection of open source models.
We’ve also partnered with Meta making Llama 2 available inside of watsonx.ai. I believe that if you look out over a five-year period, it is possible that the only source of competitive advantage in generative AI is proprietary data. If that’s true, providing model choice is actually really important because different models will be better at some tasks than they are in other tasks. And I think probably one of the most differentiated parts of our value proposition is we go to a client with a base model, it could be IBM, could be open source, we will work with them to train the model based on their proprietary data. And at that point, it’s their model.
And when I talk about some of the client examples in a minute, you’ll see in the case of financial institution that is now their model. So it’s a Truist model based on a base model from IBM with their data. And we think that puts us in a unique position in terms of helping them improve their business, but also not taking then their model and generalizing that because that would kind of compromise I would say the value proposition of working with IBM.
Last point is we are indemnifying IBM models. I don’t believe anybody else in the industry is doing that today. I know there’s been some other articles written about copyright. Copyright is actually very different from indemnification. But because we’re using IBM enterprise data, we are confident to the point that we indemnify our models to clients that are using them. I think that’s also a pretty critical part of the value proposition. So that’s watsonx.ai. Watsonx.data is about making your data ready for AI. This is an open source query engine, which is Presto and quickly moving towards Velox or Prestissimo which is the unified query engine that was born out of Facebook, and also using Iceberg which is an open table format. And I believe that will become the default for how a lot of data is served up for AI. We’re also in the process of working through a tech preview on a vector database capability which will be integrated with watsonx.data. So this is about providing all the data that you need for generative AI.
Lastly is watsonx.governance, which will be made available generally later this year. We have a lot of clients we’re working through right now on beta. For everybody that starts down this path, the minute you’re starting to get models in production, governance becomes the most critical thing. How will I explain this to a regulator? How do I understand data lineage, transparency of models? How do I explain decisions being made? So I’d say we’re optimistic on the prospects for governance as we bring that to market.
If we go to the next slide, and I’ll go a little faster here now so we can get to the Q&A. I talked about watsonx Orchestrate and using that to automate tasks. This kind of gives you a sense for what the experience looks like in the product, where you’re truly just codifying a natural language, a skill, which watsonx can then perform on your behalf. In the case of the IBM use case, we implemented this in IBM HR before the product was available. So we really use that to burn in the product. It took about a year to be clear, because we are dealing with early alpha code.
We have driven massive productivity in IBM to the tune of automating 90% of the tasks that this team was doing before. We’ve now been able to build on that capability into the product, which gets to why I’m so confident in the comments I made around ROI, because we’ve done this for ourselves. And this was automating tasks like job verification, processing promotions, job requisitions, processing salary increases, very classical I’d say white collar repetitive tasks, watsonx Orchestrate with generative AI embedded does that really well.
We go the next one or next slide please. You can then see how we would get from kind of the three major use cases I talked about to a much broader set of use cases. If you got to look at the columns, there’s one set of use cases around the customer facing experiences and interaction, then you go to I kind of call classic G&A, HR, finance, supply chain where companies are largely looking to reduce costs. Then you go to IT development and operations where as I said I think probably the biggest bang for the buck at the moment is around cogeneration. But I see this moving quickly into IT automation, AIOps, data platforming, data engineering.
Lastly is core business operations. Think of this as from cybersecurity to product development to asset management. I think these use cases will represent not the total universe, but I would say in addition to the three I talked about as the high priority ones that we’re working on, this is probably the next up in terms of how businesses will look to capitalize on generative AI and we think we’re well positioned with watsonx to deliver on these.
We go to the next slide. I’ve alluded to a few of these, but we have really good momentum in customers to date, and largely around productivity increases. Truist I mentioned talking to you about this is very labor intensive summarization that they do today around RFI submissions. Watsonx generative AI is really good at doing this. So that’s one example. Samsung SDS, delivering this as part of what they call zero touch mobility, which is really how do they deliver products faster. And again, in their case, they’re taking a base IBM model, they’re tuning it, training it based on their data, it becomes their model. They’re differentiated.
SAP, their first example or first use case, I should say, is about delivering something they call SAP Start where instead of having to know which SAP system to go into, you can just go into a natural language query box and say, show me the purchase order from this customer as an example. And they can find that right in the correct SAP system.
For those who have worked with SAP, you know that can often take a while to find what you’re looking for that now becomes seamless with watsonx powering the SAP experience. And I think NASA is an interesting one where we’ve created a unique model around geospatial data, combination of NASA data with an IBM base model, a model that we’ve actually now open sourced. And so this just gives you a sample of some of the momentum and what’s happening in the market.
And then last slide, please. This market is moving incredibly fast. I don’t know that I can give you precision on where this is going in ’28, ’29 as you look out that far, but I would think of this as more GPS coordinates, direction. This is the year AI is extending beyond natural language processing. We’ve talked about some of that. I think governance starts to go mainstream in ’24. I think as we get to ’25, AI is going to become much more energy and cost efficient when I think about how we’re doing in some of our tuning and optimization today. I think that’s very possible.
’27 is when foundation models start to scale uniquely. What I mean by that is this is the notion of AI building the AI. And that’s very different than today where we have to go through a training or tuning exercise, meaning there’s humans that are dictating the rate and pace. I think as we get out a few years, the AI starts to take over to some extent, in terms of delivering on new use cases and outcomes.
With that, Wamsi, I will hand it back to you and we can open it up however you like.
Question-and-Answer Session
Wamsi Mohan
Yes. That’s a great introduction and appreciate all the slides and delving into this so that it’s a little more structured. I guess, Rob, to kick it off maybe, I think there’s so much to delve into here. But let me first start with just the TAM, right? Like how do you think about the TAM for generative AI and what part of that TAM does IBM address?
Rob Thomas
Depending on which report you read, IDC, McKinsey, you see some very big numbers about economic impact of generated AI, 15 trillion, 16 trillion rings a bell in terms of what I read. How much of that is addressable? I would say honestly, we don’t know yet. But let me break down a few pieces. If you look at the core platform I talked about for models, I think that’s uncertain at the moment for how much — how big that market will be. For data, I think we have a pretty good feel for it. You look at the size of the relational database market, you look at data warehousing, you look at growth of data that comes with generative AI, that’s a market that is 80 billion to 100 billion, has been pretty consistently growing in that direction. Data is significant. Governance has always been a smaller market than that. But I actually think governance comes to the forefront, it probably just takes a little while longer. As you think about consulting services around this, like in many things we do in technology, we think the multiplier for consulting services is on the order of 3x. It could be a little bit more, could be a little bit less. But I’d say that’s on the order of it. As you look at kind of the assistant layer that I talked about, that’s the one that’s probably hardest to predict. Because to some extent that is changing existing business processes. So you can imagine incredibly large TAM when you think of it that broadly. I think that we will kind of learn over time how quickly that can start to take form. And then if you got to go to the bottom of what I call the tech stack with OpenShift and multi-cloud, as you’ve heard us say before, we think multi and hybrid cloud becomes the default in technology. And it’s kind of been heading in that direction. And so that too becomes a very large TAM. So I’d say we’re very optimistic about the possibility here, but it’s hard to nail down some of the specifics today.
Wamsi Mohan
That’s helpful. Well, if I was to split this a different way, Rob, maybe think about training versus inference, I know it seems like a lot of the training today is being done in the public clouds, whether it be access to GPUs or whether it’s just the inertia of learning of on-prem organizations, it feels as though most of the training is centric and public cloud. So how do you think that was or call it the next three to five years?
Rob Thomas
Certainly at the moment, there’s an arms race as we all know on GPUs for training. And logically that’s most, I’d say, effectively and efficiently done in public cloud. But if you go to some of the cases that I talked about, so we’ve invested in large GPU clusters, we’ve trained the base model. Do you need the same level of compute capacity to do tuning based on a proprietary dataset from a client? I would say not necessarily. Yes, if you have it, you can go much faster. But I’m not sure it’s a requirement, whereas with training it is kind of a requirement. It’s kind of table stakes to get an initial base model built. As you go to inferencing, our view is you can do inferencing on CPU. It does help if you have more of a custom ASIC type approach. If you look at what we’re doing in mainframe today and the AI inferencing that we do in mainframe largely for like fraud type use cases, that’s a custom chip. And you don’t need a GPU, but it is a custom chip. And so I think inferencing we’ll see how that plays out over time. But my instinct is that CPU can do a lot of the work that’s needed on inference, certainly as you get to edge type use cases as well and don’t really envision a world where we have GPUs in every edge device. I’m not sure the economics would ever make sense for that. So I think time will tell in terms of the precision of this, but that’s a general direction.
Wamsi Mohan
Okay, that’s helpful, Rob. I want to go back to one of the slides that you referenced the stack that IBM had and I believe that was, I think, a second or third slide maybe. And in there you mentioned data services and data fabric services, in particular. Can you help us think through sort of what IBM is doing specifically over here? And what sort of products that touches?
Rob Thomas
Yes. So let me just do a little bit of a distinction for a moment. When I talk about watsonx.data, that’s part of the platform. That is what I would describe as the next generation data warehouse. And if you think over 25-year period, I would say this is the start of the fourth epoch of data warehouses. First we had OLAP, then we had appliances, then separated compute and storage. So think of those as all three very different warehouse architectures. Fourth is what I’m calling the new architecture, which in our view will be completely open source, open format, Iceberg, Presto, Velox. We’re getting an incredibly high performance, meaning 2x on a separated compute storage architecture at roughly half the cost. We think watsonx.data as a next generation warehouse can be very disruptive to the market around data. Now why do you need data services? So we have that new warehouse, what’s the role of data services to your question? By definition, everybody’s data is already somewhere else. So you need a way to access that data. Think of this as traditional ETL, or data movement, bringing it to one place. What we found is the market’s more of a ELT style, meaning do some data governance, data quality, data cleansing, as you’re moving the data or after you move the data, it depends on the preference for somebody. So we’re talking about data services and data fabric. This is about how do you get all of your different data repositories acting as a single data store where you can easily extract data into a high performance warehouse like watsonx.data. And if you look over the last few years, we’ve had a lot of success with Cloud Pak for data. That is the core product behind what we’re calling data services, which is about unifying and creating a data fabric. So that teams building data science models, machine learning models, and in the future generative AI models have one place that they can pull data to serve those needs. So I think this notion of data services and the momentum we have with Cloud Pak for data is very much a part of the story.
Wamsi Mohan
Okay, that’s great. That’s super helpful. I guess I’m getting some incoming questions here from folks who are dialed in as well. Can you talk a little bit about vector database and what is the timing of that and how you can monetize it?
Rob Thomas
Nothing to announce on the timing today, but I would say in most of the companies we’re working with today, as you get down the path of building a custom model based on their data, you need a vector database capability, basically just to drive performance. There’s a lot of different options available in open source. That’s largely where we’re investing our time today. I would say it’s hard to imagine a generative AI deployment in an enterprise that is not going to incorporate vector database. It just seems to be required from a performance perspective. Now that doesn’t mean they’re not still going to have their Db2, their data stacks, their MongoDB, kind of all the companies that we partner with on other varieties of open source database. But I would say vector database certainly has a role. It’s arguably a niche-y [ph] type of role. But it certainly has a role in what’s happening in generative AI. So right now we’re kind of in experimentation mode, because of what’s available in open source, we’re able to bring things to the table. We’re thinking through productization, monetization.
Wamsi Mohan
Okay, that’s super helpful. I want to go back to your comment, Rob, about in a five-year period like the true differentiation might just be proprietary data and sort of models might really not — I mean, models can be generally available and that’s not going to be the source of differentiation, per se. Can you clarify a little bit about in the use cases where you have used foundation models in conjunction with clients on data, how much of a speed up has there been in sort of timing relative to someone who’s trying to start from scratch and do this? So what is the kind of time to market advantage? And maybe in your Truist use case, how did that come about from a consulting standpoint? And what was the involvement of that? And kind of maybe how long did it take just to put some numbers around that would be helpful?
Rob Thomas
I believe the start from scratch market is relatively small, meaning it’s probably the 5% to 10% of the use cases we are doing some of those where a particular company has a very unique need and it’s best served by start from scratch. But the reason I think that market’s pretty small is starting from scratch entails all of the investment that building based models did in the first place, because you’re starting from scratch. So if you think about what I talked about how we were at this three years, those are the handful of companies that want to invest two to three years before they will ever have something come to market. I’m not saying there’s no market there. I’m just saying I think that’s relatively smallish. I think for most companies, their needs can be met by a base model, whether it’s from us or from open source. We’re kind of open on how we do that. We’ve done projects that leverage Llama 2. We’ve done projects that leverage Hugging Face models. We’ve done projects that leverage IBM models. It’s really about you have a toolbox and you’ve got a hammer, you got a screwdriver, you’ve got needle nose pliers, you got nails, you got to figure out what is the best tool for the task at hand. As we look at IBM consulting around this, what’s interesting about generative AI not unlike cybersecurity is in the IT world we actually work on very few things that become a board level topic. Cybersecurity was the first one, generative AI is the second one. I can confidently say those are board level topics. That’s why it is a benefit to us at IBM having some like IBM consulting, because when you have something that’s a board level topic, it becomes a question of how do we drive this as part of the business transformation? Do we have the talent we need to do this? Can we do change management? Do we have the project management we need to deliver the program? So having IBM consulting as part of our go-to-market motion, not exclusively, we do work with all the other GSIs whom we’re establishing centers of excellence with as well. I do think the role of an SI is important for generative AI. And when you think about the three use cases that I talked about, the ones I said are proven, high value, those are ones that we’ve really learned that in IBM consulting engagements since the start of the year. So I’d say very optimistic about the combination of consulting and generative AI, but I’d say equally bullish on — I’ve met with all the major GSIs on this topic in the last three to six months; Accenture, Deloitte, EY, Wipro, HCL, TCS. You name it. We’re actively building practices with them around watsonx.
Wamsi Mohan
That’s great. That’s super interesting, Rob. So going back to your three proven high impact use cases, right, like the HR example, the conversational AI example and app modernization, where would you — if you think about it through the lens that there was some level of productivity that consulting was helping with to begin with or our app modernization that they were helping to begin with, what is the incremental opportunity here versus what is this like using generative AI as a toolkit to enable that productivity improvement. I think people were doing productivity based projects now for a little bit of time. Now maybe generative AI just helps them get there faster. But does it also support incremental dollars from an IBM perspective?
Rob Thomas
One is certainly increasing cycle times in terms of time to get in live and getting successful. And kind of the customer service example we’ve talked publicly before about how NatWest has been using watsonx, they white labeled it. So effectively, they have their own name for it. And the difference is when you bring generative AI to this, the accuracy improves much faster. So as I think back a few years, it took us — the first went live with NatWest, we were like 30% containment, then we got the 40%, then we got the 50%, then we got the 60%. So it was kind of a classic machine learning, deep learning problem where you’re iterating you’re making progress as you go. With watsonx Assistant, we can get to 60%, 70%, 80% way faster. And then it’s about can you get up into the 90s. And that’s where I’d say the real breakthrough happened. So I think it’s cycle time. I do think there’s an increase in wallet share too, though. Code Assistant is a brand new capability. We weren’t even playing in the market of Code Assistant before watsonx came to the forefront. So to add modernization, that’s almost I’d say all incremental because we weren’t really playing there. Yes, we’re doing application modernization. That’s still needed, still part of what we do on our consulting practice. But bringing something like Code Assistant on top of that, it gives a client greater incentive to build more applications in them. So somebody that’s using Ansible a little bit now, the odds that they’re going to then invest in more Ansible we think is much higher when Ansible developers are way more productive using watsonx Code Assistant. In the case of some of the talent use cases, I think this is very different than RPA. I think everybody that’s been through RPA projects, they understand the benefit of a rules based system. But there’s very little that actually happens back in the source systems. So that meant that you can do something at the application layer with generative AI and it’s also populating the source systems. To me, that means companies are going to be much more open to doing that, because then you’re actually implementing use cases into their existing architecture. So I do think this represents in speed time to market, time to value for clients, but also incremental upside for IBM.
Wamsi Mohan
Yes, that’s super interesting. Rob, just on the Code Assistant, like how broad based are the applications and do you intend to have subsequent generations that become more broad based? Obviously there’s different Code Assistant out there with sort of like GitLab, GitHub frameworks, whatever you be. But from an IBM perspective, as we think about the roadmap for this, because it does seem like it is a very obvious like productivity enhancement use case and you’re talking about now very high quote acceptance rates, which is quite amazing in the environments in which you’re targeting and running, how broad based can this become?
Rob Thomas
We’re supporting 100 plus programming languages in our model today. We have announced tech preview general availability of the ones that we think have a lot of momentum and product market fit today. So Ansible and then for mainframe, but I would say this is just the start. We are encouraged by early signs on how this generalizes to the other programming languages. The main way I think about timing is just as to your point, code acceptance. As we get to higher levels of code acceptance where we want to release that because we think there’s been an opportunity to monetize that. So I would say stay tuned as we go. But if you think about that assistant layer that I talked about, if I look out a few years I envisioned us having 10, 20, 30 assistants. I could imagine a lot of different variants where as we start to do more use cases, we see commonality of use cases, we deliver a whole family of assistance, and there’ll be a number of those in code specifically.
Wamsi Mohan
Yes, that’s super impressive. Can you talk a little bit about maybe I think you just mentioned sitting with board level execs and CXOs to talk about AI and generative AI, are clients talking about any impediments? What is the hesitancy? What are some of the concerns maybe around governance or data or skills?
Rob Thomas
Number one that comes up for everybody is where’s my data going to go if I do this with you? I think we have a great answer for that. So I actually welcome that question. Because if you’re working with IBM, your data is going nowhere. That becomes your model. And it doesn’t inform any other model, it’s not going to get generalized in a way that you’re helping your competition or anybody else. So that’s a common question. Second is will IBM stand behind this? Do you have my back? To my point on indemnification I think that is why that’s a key point of our value proposition is indemnifying and standing behind our models. That’s a common question. Third is I’d say even broadening the point on governance, which is why I’m pretty excited as we get towards year end and deliver watsonx.governance, because topic of governance is way beyond do I understand who’s accessing the data. It’s data lineage, it’s data provenance, it’s model drift. If my model starts to get very different answers over time, how do I understand that and course correct. And I think governance is not interested to anybody when you’re not in production, the minute you’re in production, it suddenly becomes like oxygen, which is I can’t imagine being in production and not having this. So I think that becomes a pretty critical piece over time for us. But it does come up in every discussion early now. But it’s not really where people start. Because they want to start with well, I need to get something headed towards production, something working, some type of ROI, the use cases that we talked about. At that stage, I think governance becomes very important.
Wamsi Mohan
Yes, that makes a ton of sense. We’re coming up on time here, Rob, and there’s so much to talk about, but maybe to wrap up, Arvind compared the AI opportunity to kind of Red Hat adoption. What would you say around the traction in the business, anything you can talk about from a pipeline standpoint, what’s happening to the opportunity set? And off the different elements that you touched on, including — you have this great slide on all the use cases, any particular ones in there that are seeing better traction than others in these early days?
Rob Thomas
Kind of the Red Hat analogy was around building deep technical skills in IBM consulting to ease adoption. Obviously, this is different from Red Hat in one respect in that Red Hat was an existing business. This is greenfield. This is all a new business. But then to some extent, that puts an even bigger impetus on skills. And the big point as we have started going to market aggressively really since January, we measure a number of pilots that we’re doing in IBM consulting, client engineering engagements, where we’re actually delivering a specific MVP. And we’re seeing really good traction in terms of volumes, outcomes, what we’re able to deliver, so I’d say stay tuned, but very optimistic in terms of the interest and what’s happening here. On the use case, I don’t think I have anything more beyond there’s clearly three lead use cases that we talked about. The other longer list I think time will tell where did those really gravitate to? But I wouldn’t be surprised if automating the next G&A functions is not towards the top of the list. I think that’s high odds. And I think more around IT automation how companies run their IT systems. I think both of those are high odds. Last piece I mentioned on that is since you and I last spoke, we closed the Apptio acquisition. I think the missing piece to the puzzle for us on IT automation was financial operations. How do you actually bring the financials to what you’re doing in your IT? So really excited about Apptio. We now have $450 billion of anonymized IT spend, which as you can imagine could plug into large language models over time. So really excited about Apptio and what we’re doing there as well.
Wamsi Mohan
Yes, absolutely. Congrats on closing that deal a little bit earlier than expected. And, Rob, thank you so much. This was super helpful. Really appreciate your time and walking us through this once in a lifetime opportunity.
Rob Thomas
Thank you, Wamsi. Great to be with you.
Wamsi Mohan
Thanks.
Read the full article here
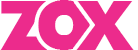